Machine learning has changed the world around us in more ways than we can imagine. Lurking under the shadows of artificial intelligence, it has emerged as one of the most distinctive fields in today’s times. Not only is it solving one of the greatest problems in history but also doing in the least amount of time that we’ve witnessed to date.
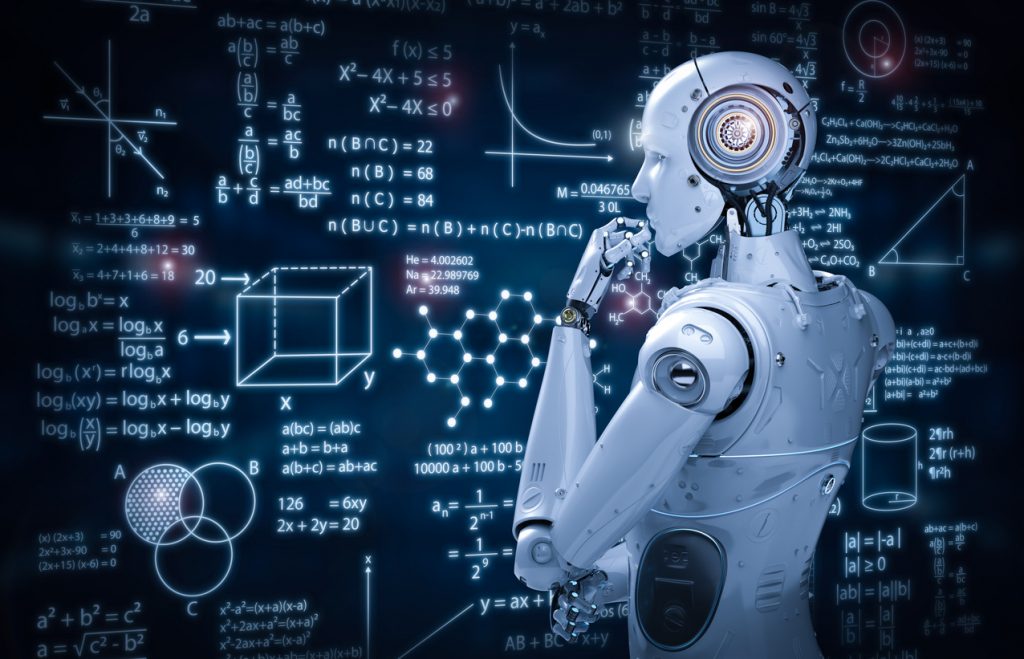
If you think about it this way, many researchers have dedicated their entire careers coming up with bases on which actual projections related to a problem could be made. Years of rigorous mathematical calculations and people come up with something commendable. But with machine learning in the picture, all one needs to do is start looking at a problem from the raw data and there you go!
Modern Software Testing
Take a look at application software from today’s generation. They are one of the most varied categories of software you can ever come across. For starters, they are running on different platforms like mobile, desktop, personal computers, tablets etc. Moreover, there needs to be integration between these devices so that the user data is retrieved and coherency is maintained. Adding to this is an emerging set of devices such as wearable smart watches, home automation appliances, autonomous cars and more.
All of this makes the sustainability of modern application software quite challenging. Furthermore, with emerging technologies like the Internet of Things, not only is the range of devices becoming large in the world, but their connectivity remains one of the most important issues. The scenario of cyber threats is already known to everyone, with businesses and enterprises losing millions of dollars each year due to vulnerability in their application.
Even though the safety and well functioning of a device is as much dependent on its hardware as its software, it all comes down to good practices one adopts in software testing services. Taking a look around, we come to learn it as agile methodologies.
Agile practices are being implemented in the software development lifecycles these days with a lot of rigor. Even though it comes down to the effectiveness of leadership, organizations all over the world are realizing its importance. This is making the global automation market grow rapidly and even more fierce than ever before. Statistic suggests that the global automation software testing market is expected to grow from USD 12.6 million from 2019 to an astonishing 28.8 billion by 2024. This demonstrated a compound annual growth rate of 18 percent during the forecast period.
But agile practices have taken software testing services to an entirely new level. Take DevOps for an example. It is taking both the test volume and complexity to unparalleled heights. In fact, one of the world’s largest airlines runs 1.3 million test executions for every version cycle, in a complex, multi-facet environment. This covers multiple types of tests, frameworks, engineers and other resources in the process.
Moreover, implementing the results of so many test cases in no child’s play. It is difficult for even the most dedicated and skilled people. In spite of the fact that they have embraced automated continuous testing to a greater or less extent, in many cases it is not sufficient to implement test automation in a reliable, efficient and authentic way through the DevOps pipeline.
If you’re having trouble understanding it, this will put the context straight. Statistics suggest that more than 40 percent of the failed automation attempts were a direct result of scripting issues. This ultimately undermines the value of the CT. If you take a closer look, scripting is one of the biggest causes of automation failure. But the problem is that without automation, DevOps is hard to achieve distant reality. And that’s where machine learning comes into the play.
Machine Learning In Modern Software Testing
Coming down to the latest tools and techniques for automated testing, machine leaning tops the list. When combined with the power of data analytics, machine learning makes its way to the root cause analysis toolbox. It is the right tool to solve the existing challenges of the agile methodologies and take it a notch higher to perfection.
Quintessentially, machine learning can eliminate the need to write and maintain test scripts. Instead of writing and correcting what goes wrong, the scripts are learned by the machine, so the changes are made agnostically. This means that the scripts continuously run and heal themselves. And they do it without getting in the way of the developmental pipeline.
While one may not be able to distinguish vividly the applications of machine learning in desktop applications, they are easily reflected in mobile and web app testing. Being complex and fragmented in nature machine learning has a lot of scope in this area and helps people understand testing, in much simpler ways than before. Ml cannot just keep these platforms connected but also make them visible and in a ready state mode.
In yet another way, ML shows trends and patterns, not only helping to visualize all the data but also provide further insight and make sense of what has happened over a certain period of time. For example, it can help identify one of the most problematic areas in an application which gives the quality analysis teams a great deal of depth to their software testing pipeline. Ultimately, it helps teams save time and measure actual impacts so that they can work on improving the strategy and other aspects of testing in each consecutive cycle.
Conclusion
Software testing isn’t a process to be considered towards the end of the software development life cycle. It has to start right from the development stage for the best results and sustainability for the product in the market. With existing challenges in the industry and faster deployment of products, it becomes essential for the leaders to adopt machine learning and harness its potential for greater efficiency and detailed identification of bugs.